"To understand something, write about it," they say. I'm curious. So I started writing: first on my personal blog, mostly about data vis when I got into the field around 2014. There was so much to learn! Since November 2017, I get paid by Datawrapper to do just that: Figuring things out, and taking you all on a tour with me. These are my best articles from both eras:
Hi! My name is Lisa
.
I create, talk & write about data vis, currently for Datawrapper in Berlin. Here I show what I've worked on in the past few years.
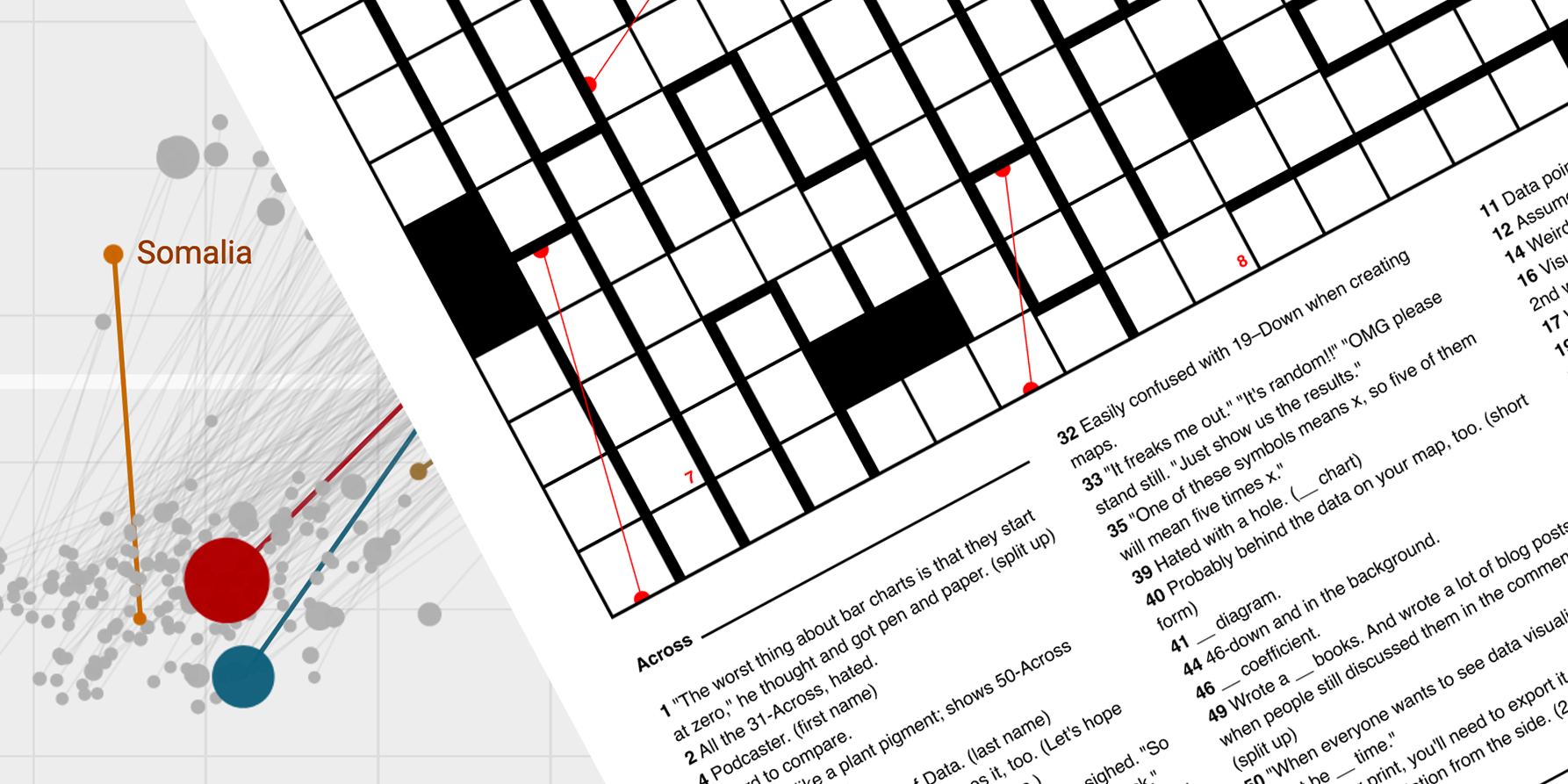
A data vis crossword puzzle!
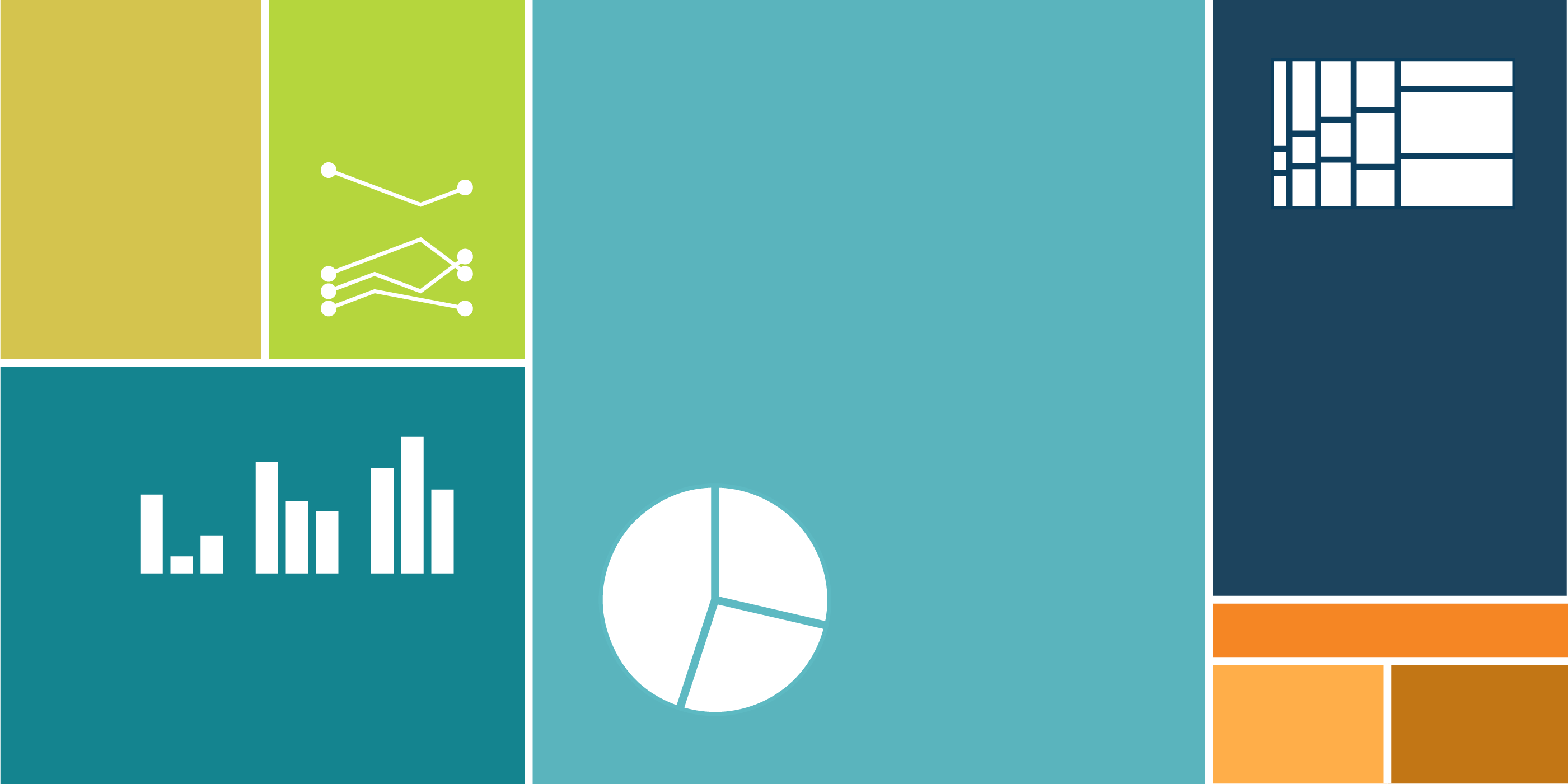
How to pick more beautiful colors for your data visualizations
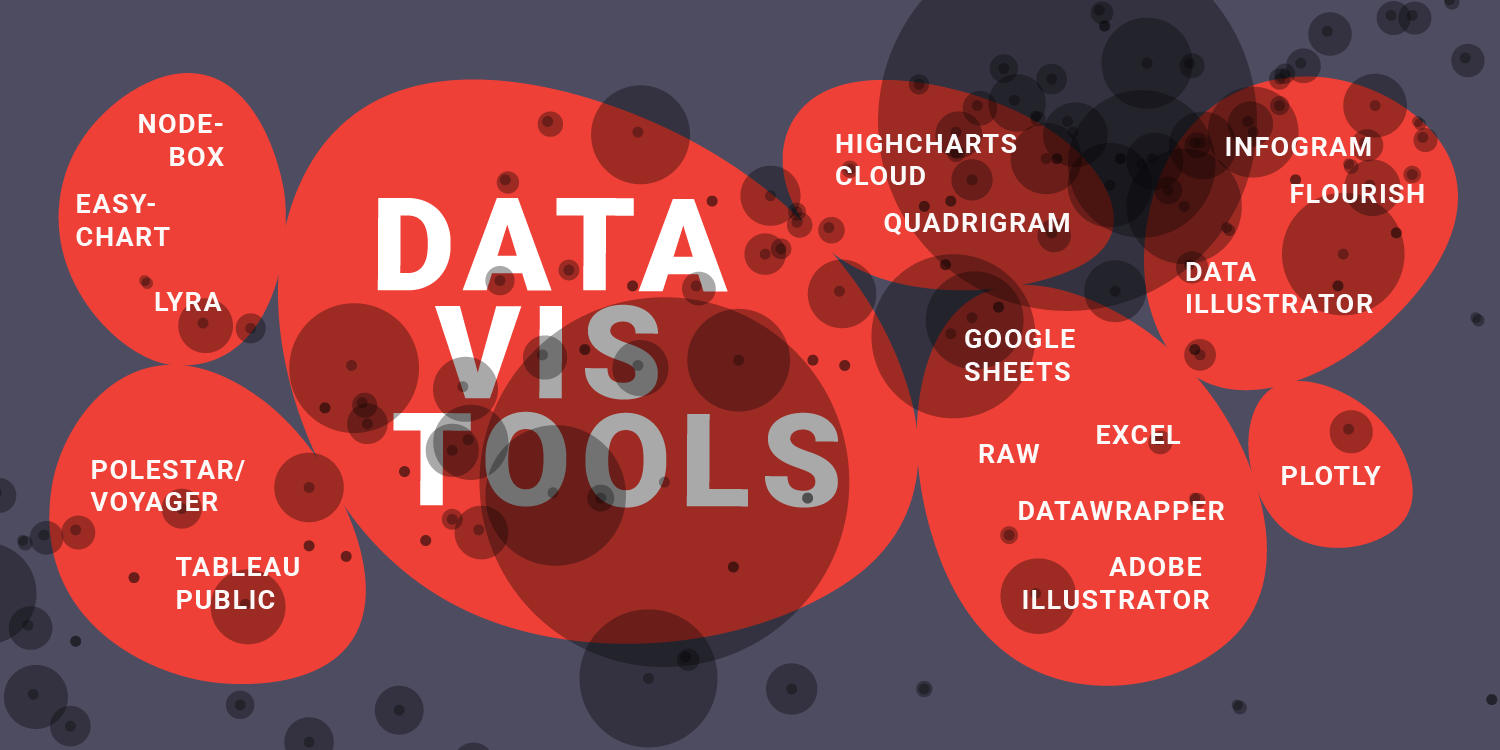
One Chart, Nine Tools – Revisited
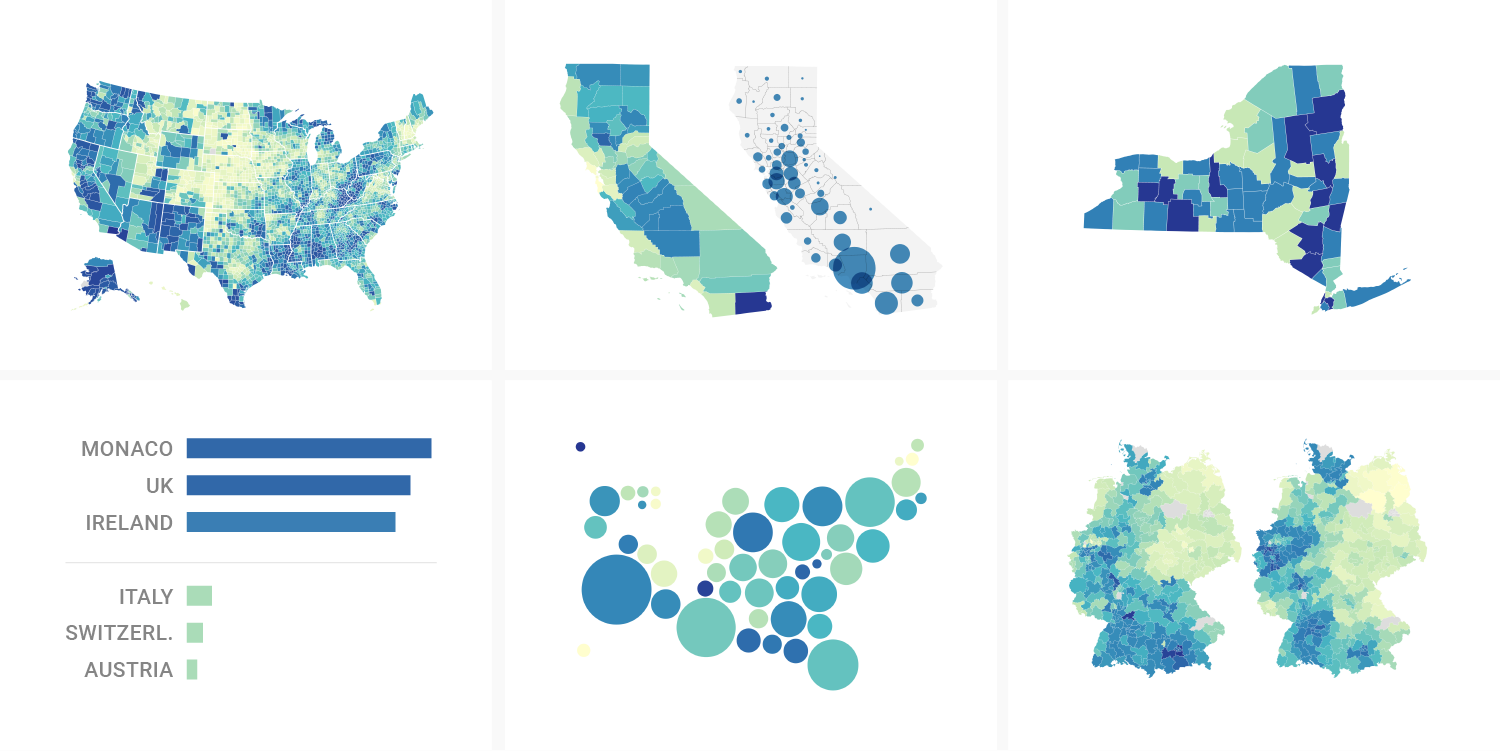
What to consider when creating choropleth maps
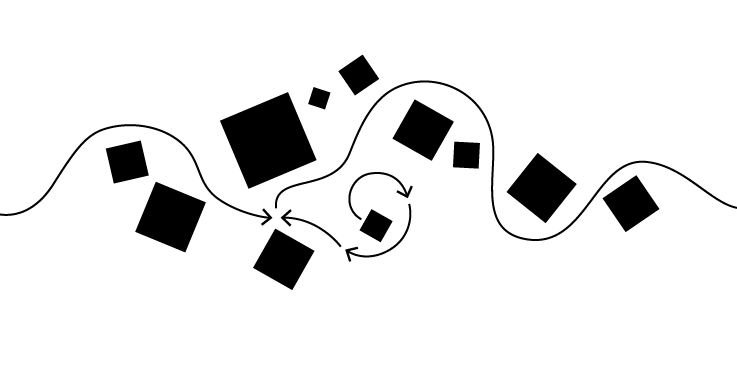
Embracing Curiosity
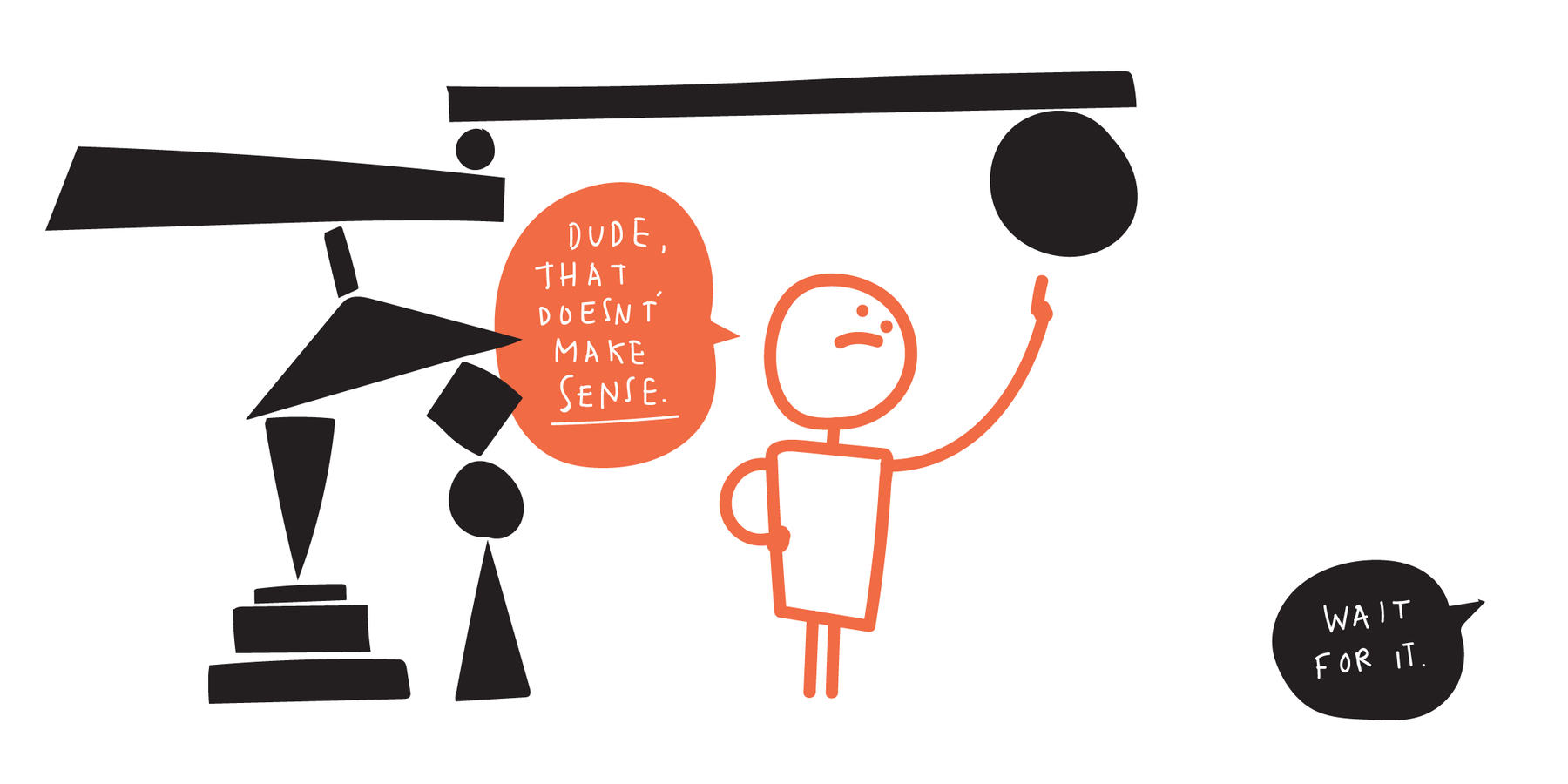
How I Feel When I Learn To Code
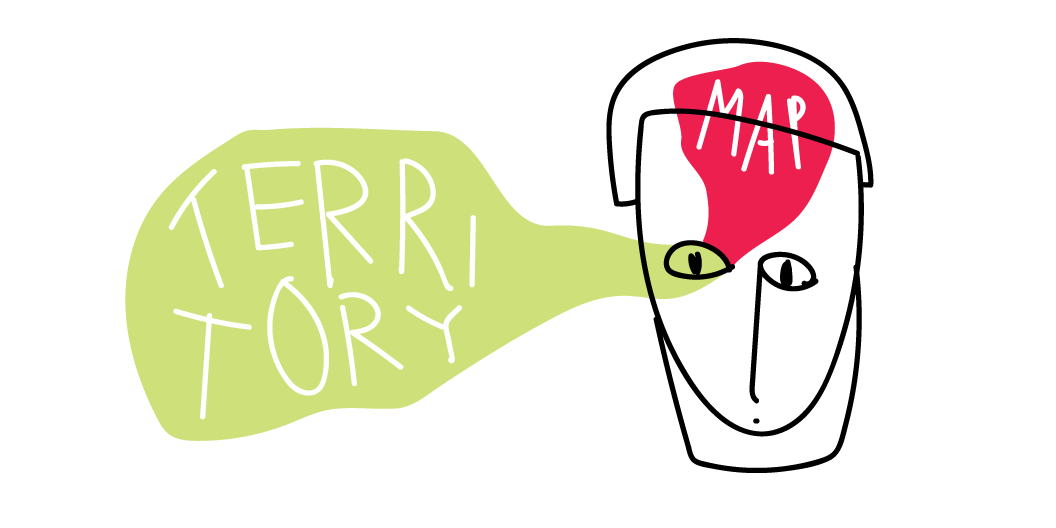
Map vs. Territory
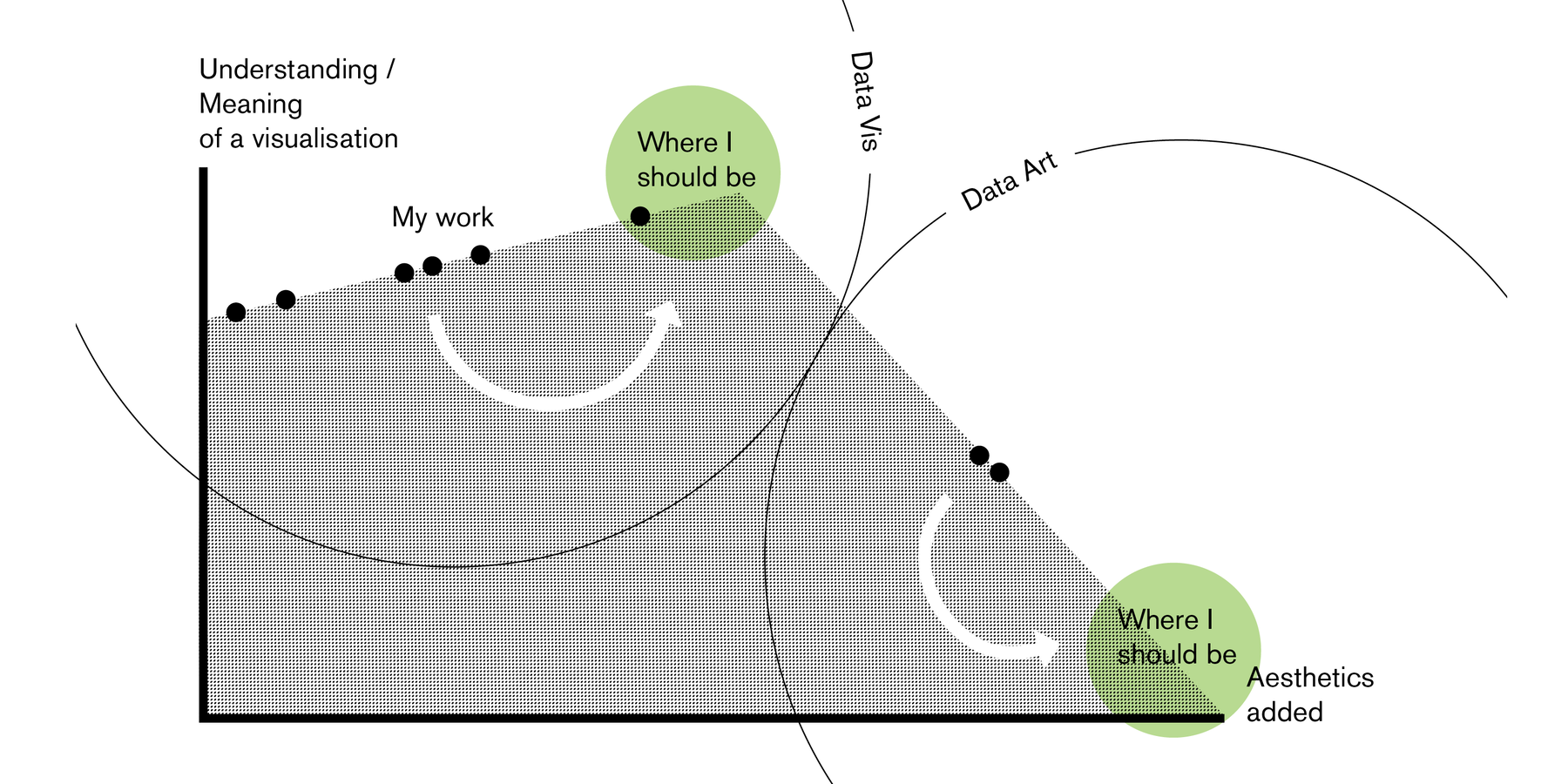
Meaning + Beauty in Data Vis and Data Art
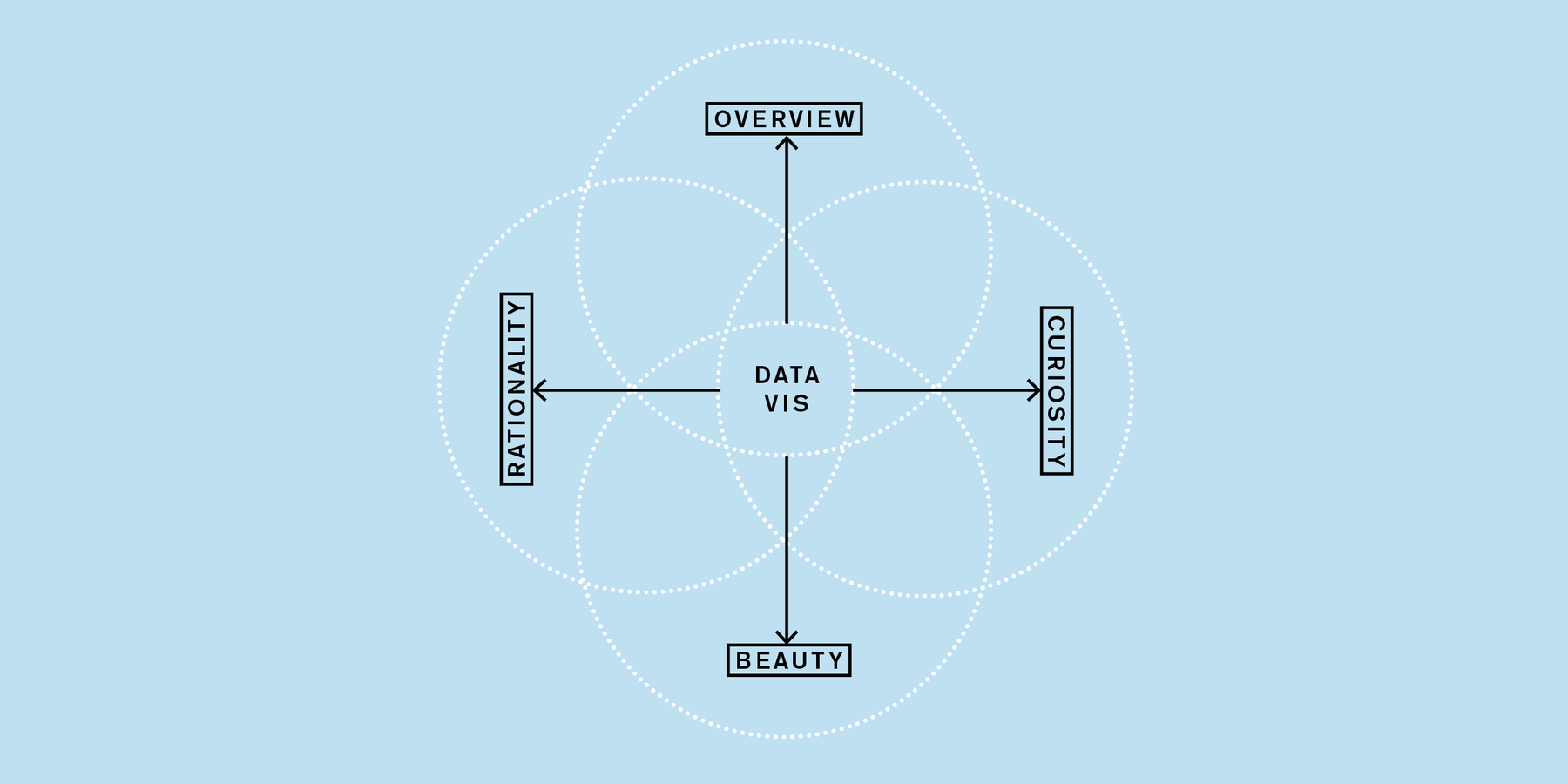
Personality Traits for a Data Vis Career
Selected Talks
Talks let me combine images (slides) and text (my speech) in a more tension-building, direct way than blog posts. I taught data vis at different universites and have given general data vis workshops over the years – but here you can find the talks I used as an excuse to research a completely new question:
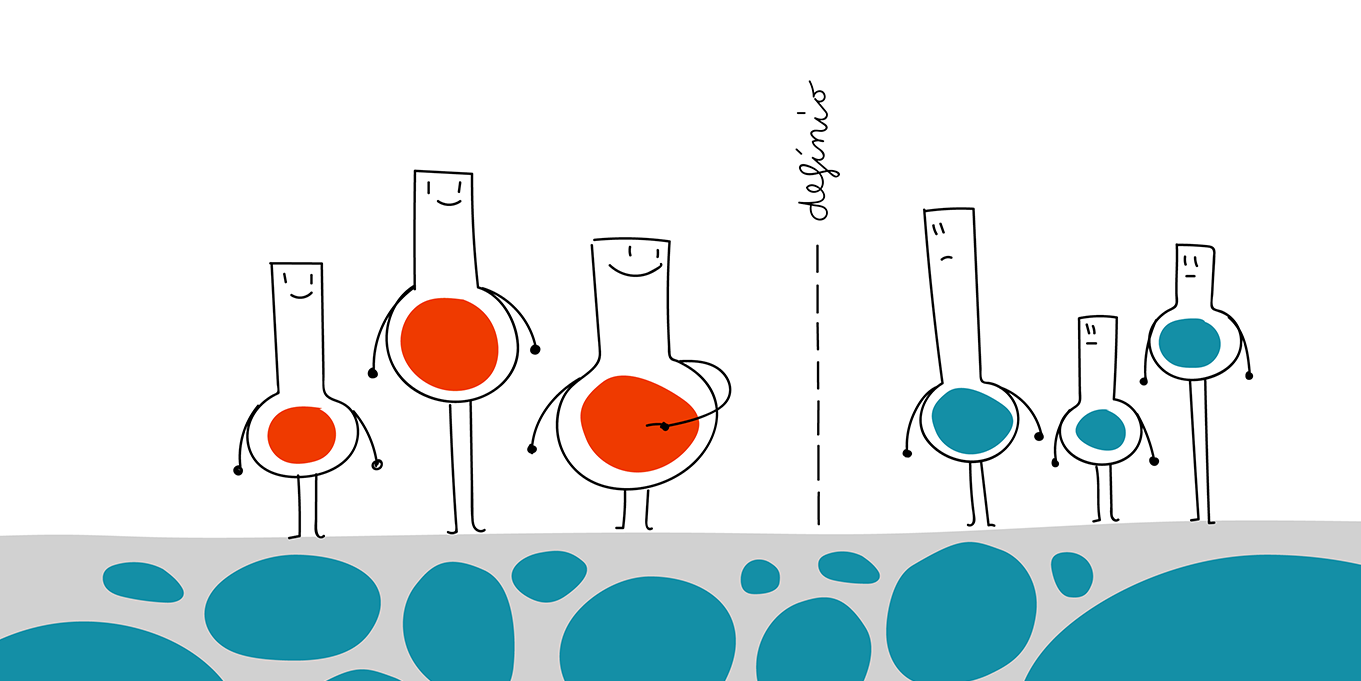
Why We Don't Believe In Facts, And How To Fix That
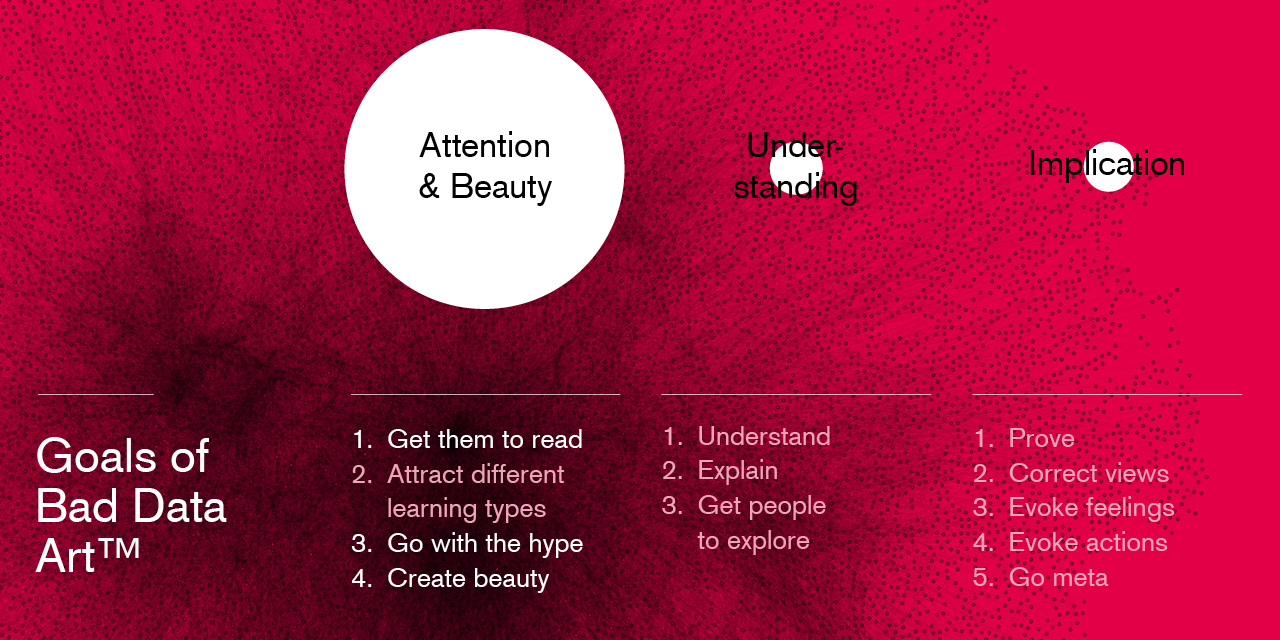
Why do we visualize data?
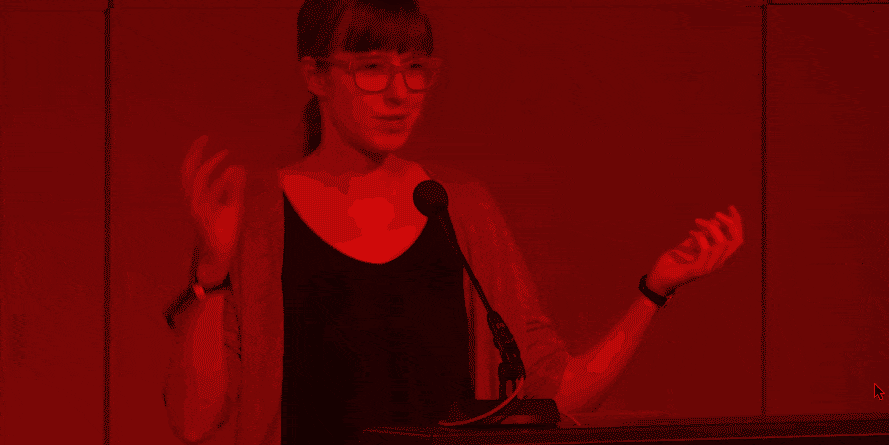
A Data Point Walks Into a Bar
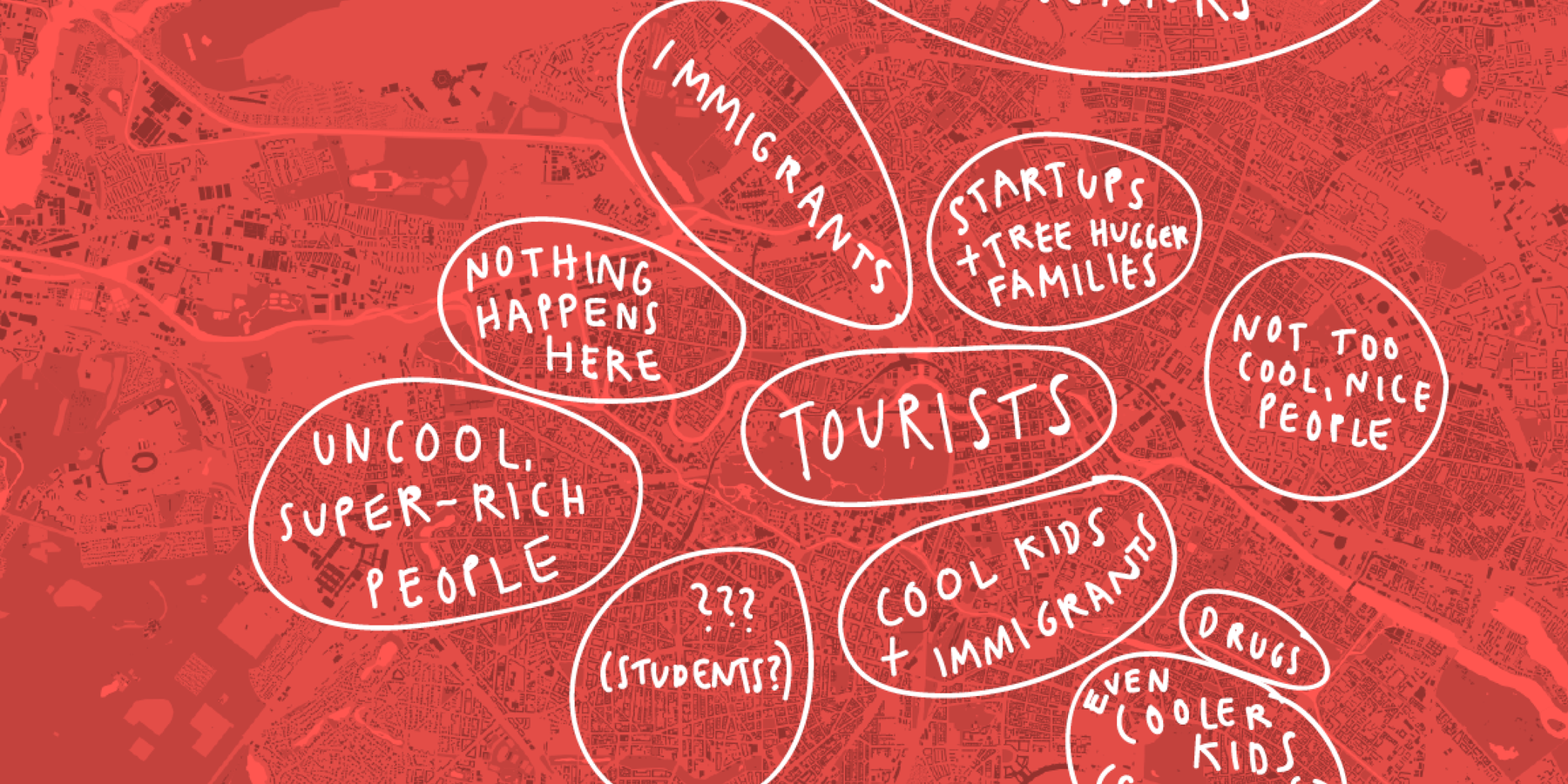
Map Poetry
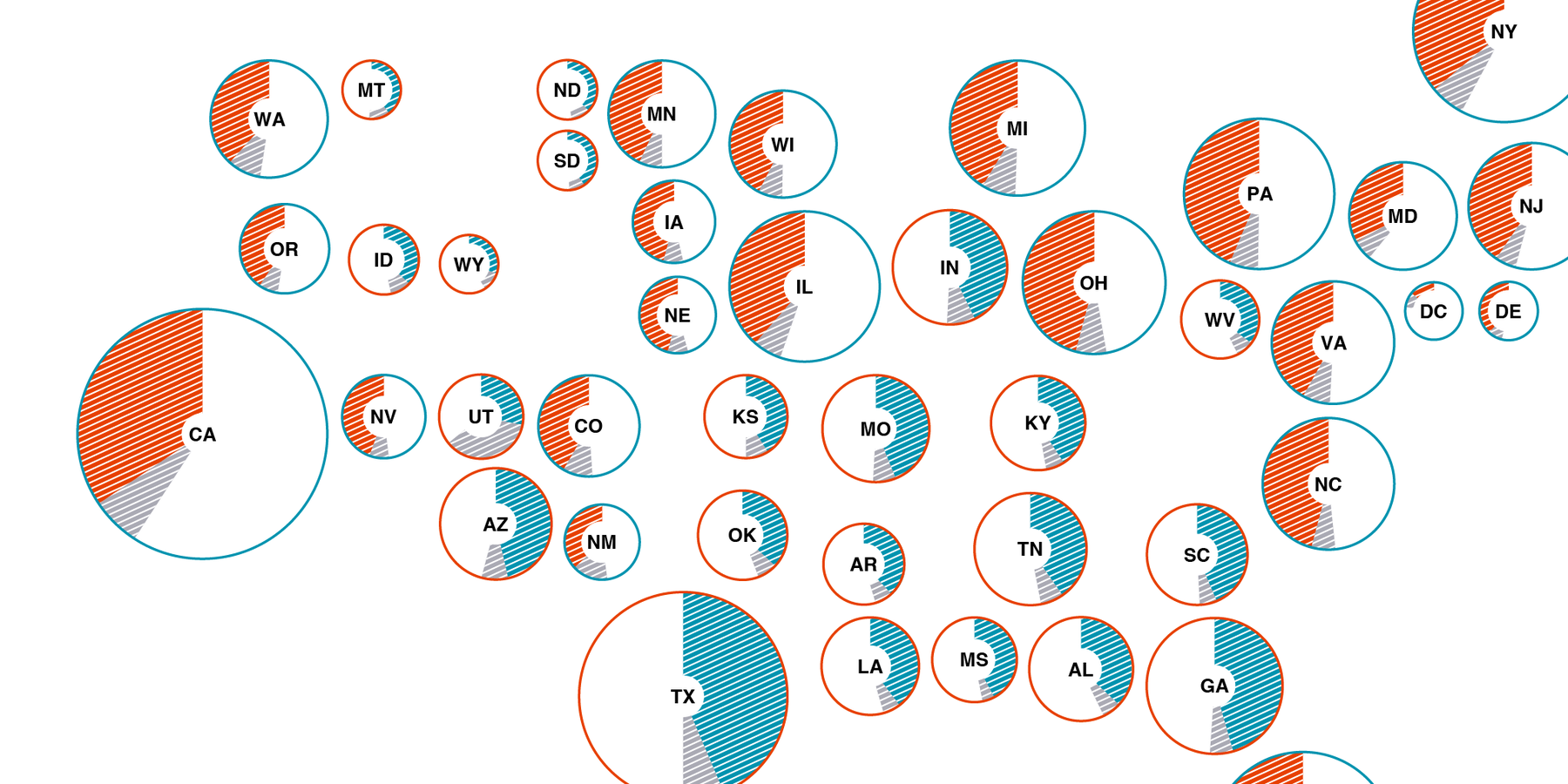
Making Election Maps Popular Again
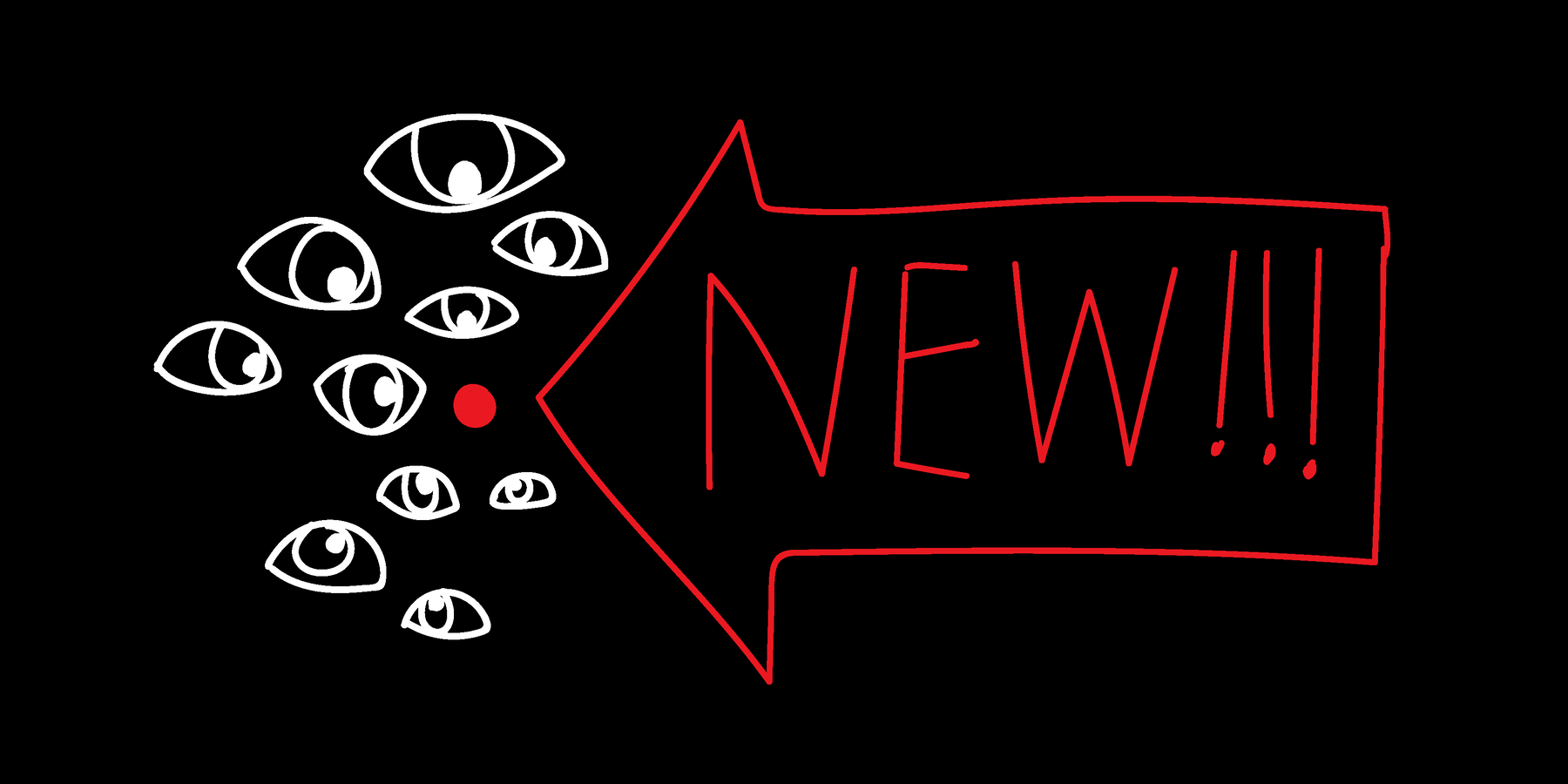
Less News, More Context
Selected Projects
Before delving into data vis, I studied print design for six years. These are some of older student works (still data vis related-ish), and newer ones I've created for newsrooms or my blog. You can also find some charts I've created during my time at Datawrapper:
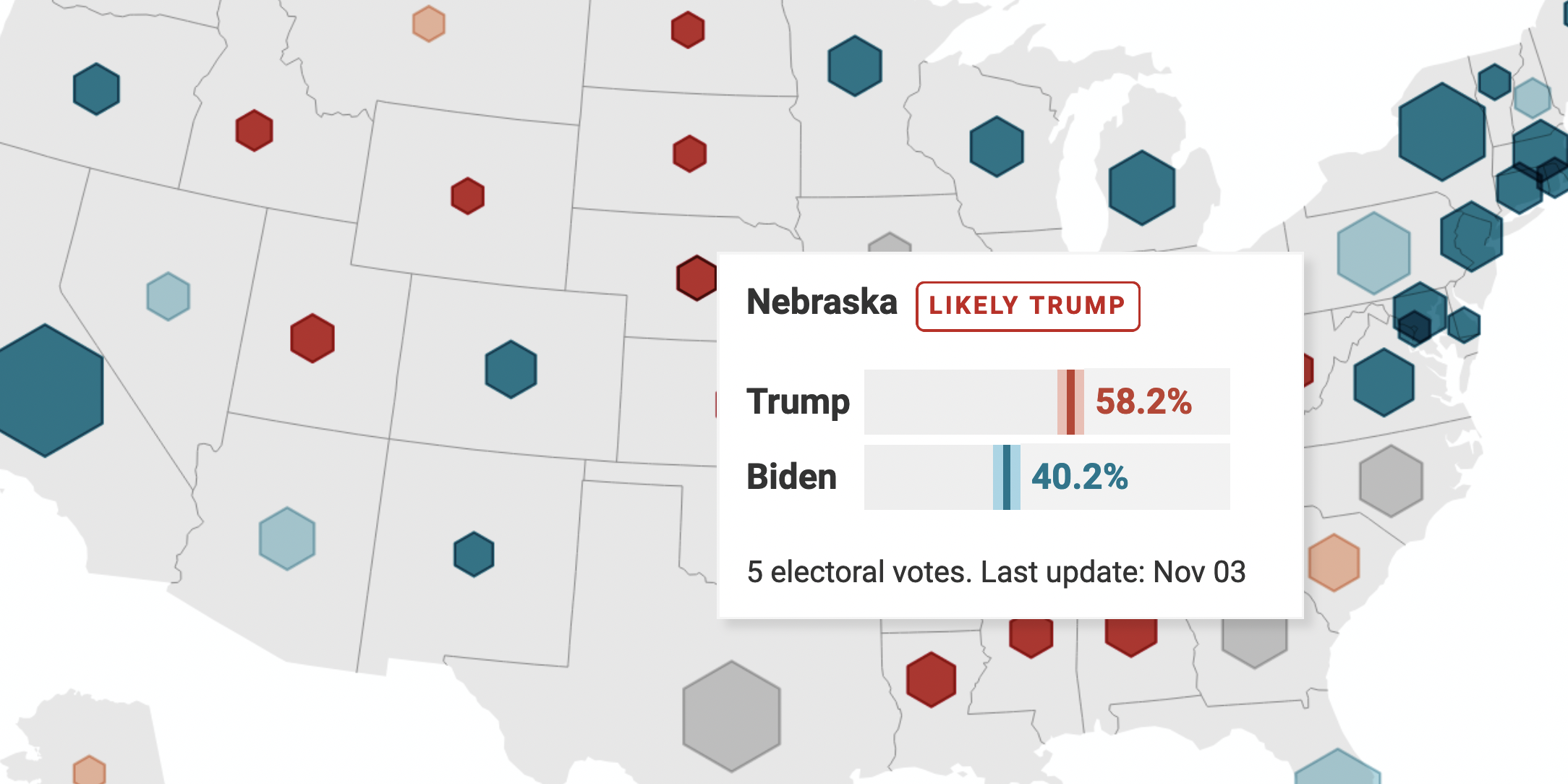
Datawrapper visualizations I created in 2020
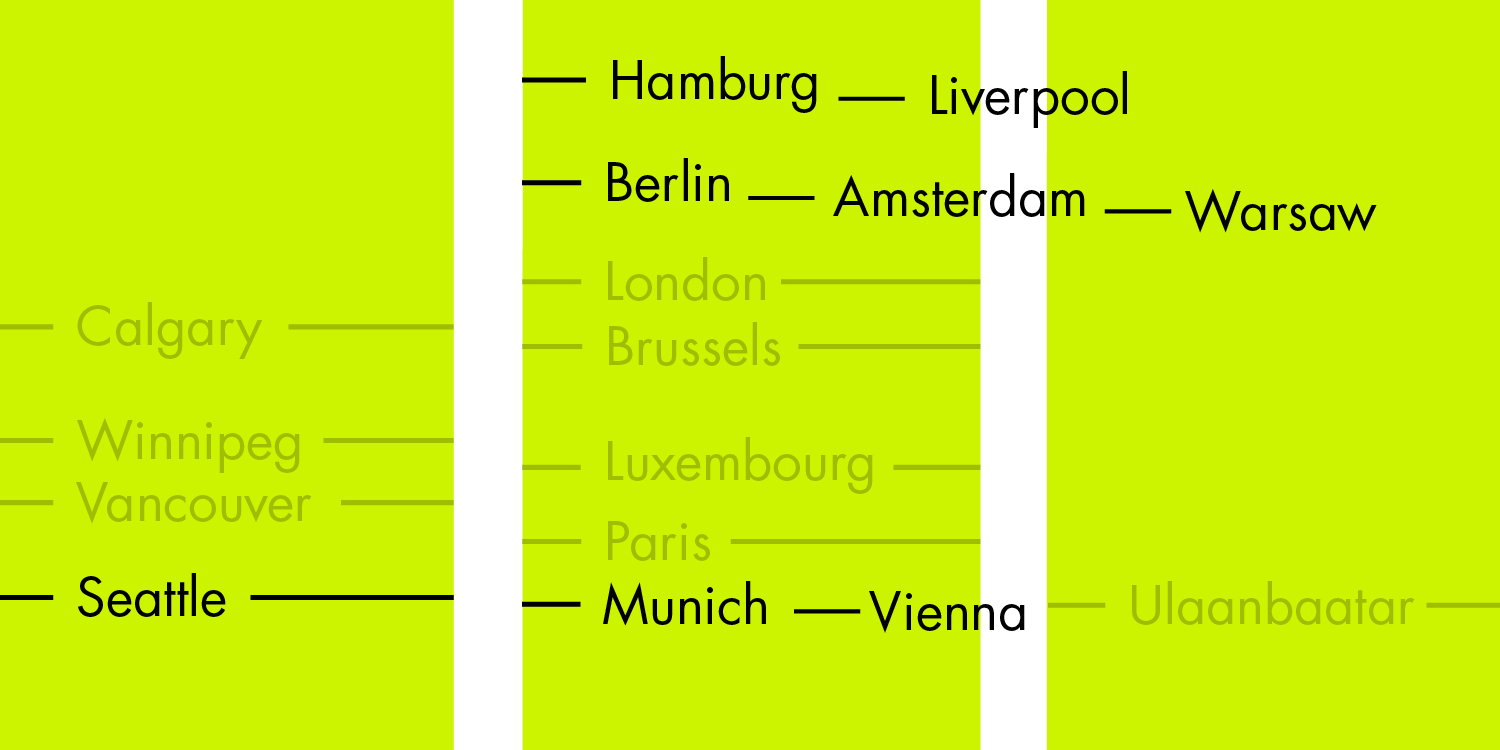
Which Cities Are On Similar Latitudes?
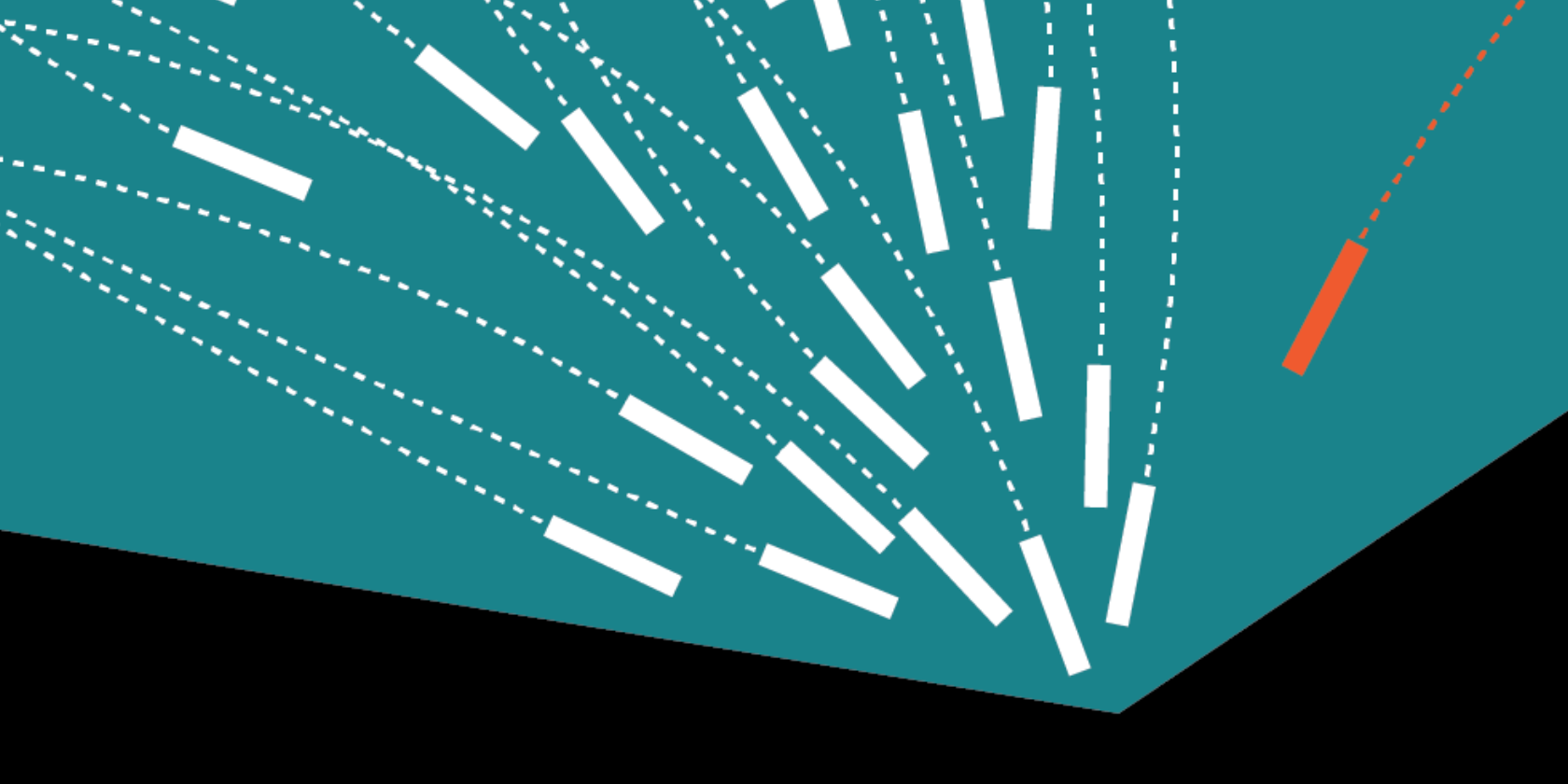
Back then, everything was worse.
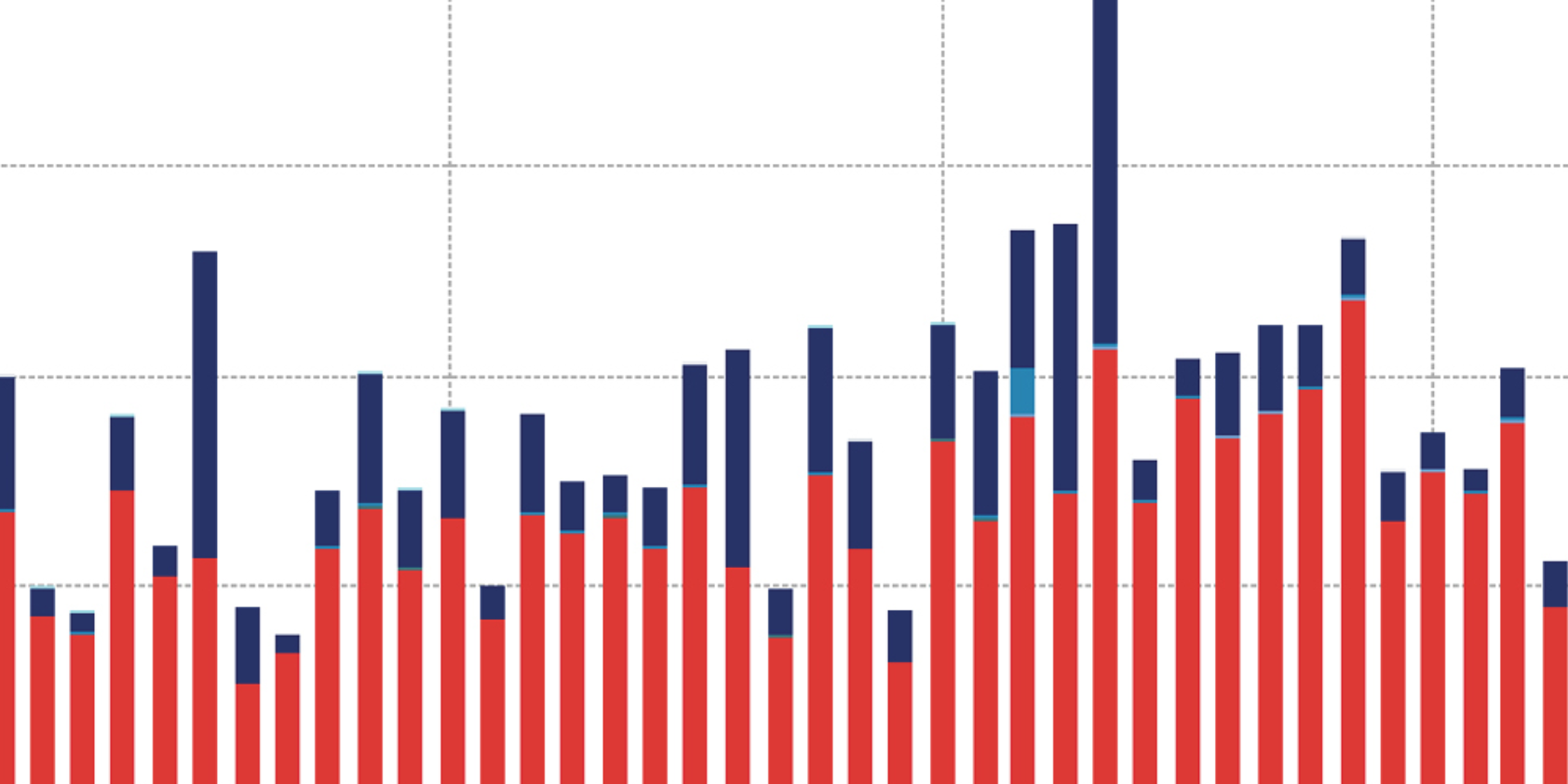
My Google Search History – visualized

Data Vis in my Master's Thesis
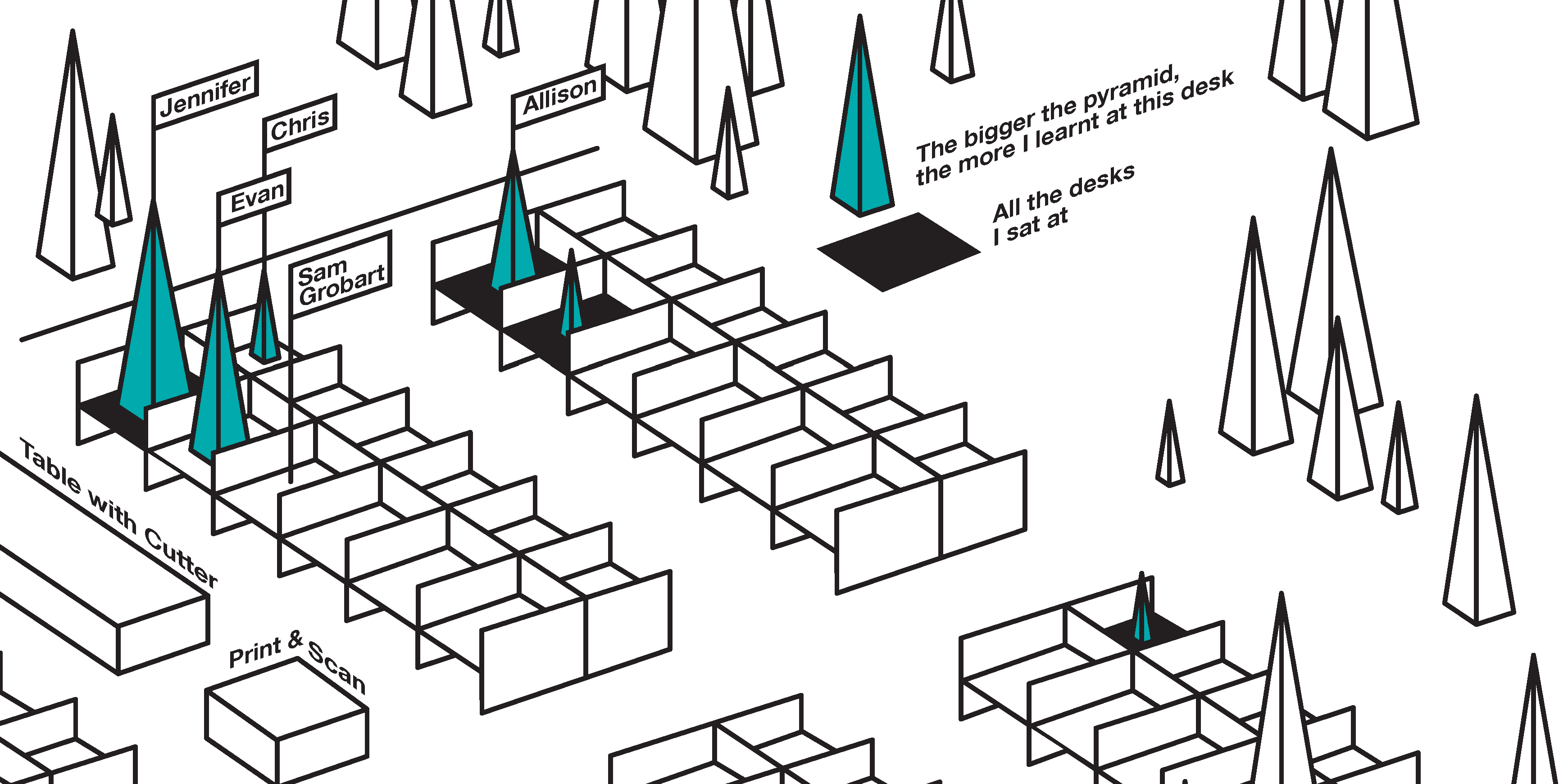
Documentation of my internship at Bloomberg Businessweek
About me
Lisa Charlotte Muth is a Berlin-based writer for the data visualization tool Datawrapper. Before that, she studied graphic design for six years and created data visualizations for newsrooms like Tagesspiegel, Bloomberg, SPIEGEL, ZEIT Online, and NPR as an OpenNews Fellow. She co-hosted the Berlin Data Vis meetup, started the global, digital Data Vis Book Club, writes a handbook, and tries to live a good life.